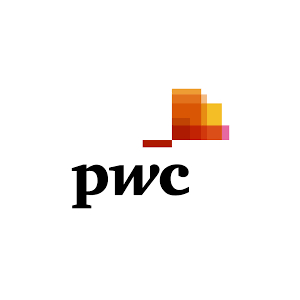
“Once again Saltmarch has knocked it out of the park with interesting speakers, engaging content and challenging ideas. No jetlag fog at all, which counts for how interesting the whole thing was."
Cybersecurity Lead, PwC
In a world where AI technology is shaping our future, we delve into the emerging trend of "small" large language models (LLMs) such as Vicuna, Koala, and Alpaca. No longer reserved for tech giants, these LLMs are making advanced AI capabilities accessible to everyone, running on common hardware like laptops and even web browsers. We explore the impact of this democratization on various sectors, the potential it unleashes for individual developers, startups and medium-sized businesses, and how it's reshaping the landscape of AI and technology at large. We'll also discuss how the advent of these models is contributing to a wider AI safety and regulation discourse.
Large Language Models (LLMs) have revolutionized a host of applications, from text generation and language translation to creative content authoring. However, their large scale has traditionally necessitated significant computational resources, making them an exclusive preserve of well-funded organizations.
Recently, a trend towards smaller, more efficient LLMs like Vicuna, Koala, and Alpaca is democratizing this powerful AI capability. These 'small' LLMs offer similar functionalities to their larger counterparts but with greater efficiency, rendering them accessible to a broader audience including individuals and small enterprises.
The evolution of smaller LLMs is propelled by several drivers:
Thus, the advent of small LLMs is a pivotal moment in AI, broadening its reach, and stimulating the potential for unique applications.
1. Vicuna Vicuna is a versatile, open-source chatbot trained on user-shared conversations from ShareGPT. It is adept at responding to varied prompts, making it applicable across customer service, education, and entertainment domains. Learn more about Vicuna
2. Koala Koala is an affordable dialogue model trained on a mix of public datasets and large language model dialogues. It excels in text generation, language translation, creative content writing, and providing informative responses. Additionally, Koala has an impressive capability to follow instructions and complete tasks. Explore more about Koala
3. Alpaca Alpaca is an instruction-following model, currently under development, trained on data generated by OpenAI's text-davinci-003. It can follow instructions and execute tasks like writing emails, crafting presentations, and managing social media accounts. Alpaca is expected to be a valuable asset for researchers and developers. Discover more about Alpaca
Small LLMs have several capabilities that make them attractive for a variety of applications. These include:
Efficiency
Accessibility
Functionality
As small LLMs evolve, they're paving the way for more innovative and unique applications. Here are a few compelling use-cases:
1. Customer Service: Small LLMs, employed to develop multilingual chatbots, can enhance customer service by providing round-the-clock support and lowering the cost of human customer service representatives.
2. Education: They can aid in building educational applications that simplify concept learning for students, thereby enhancing accessibility, affordability, and personalization in education.
3. Creative Applications: Small LLMs can underpin creative applications that generate text, translate languages, or answer questions innovatively. This can open new horizons for art, literature, and entertainment.
4. Research: These models can assist researchers in analyzing voluminous datasets and generating fresh insights, accelerating the research pace and paving the way for new discoveries.
5. Business Intelligence: Small LLMs can furnish data-driven insights, aiding businesses in making informed decisions. This can enhance efficiency, profitability, and customer satisfaction.
In summary, small LLMs are empowering organizations to accomplish more with less, aiding in task automation, personalizing customer experiences, facilitating informed decision-making, and fostering quick innovation.
Developers and businesses are capitalizing on the capabilities of small LLMs for a plethora of applications:
1. Google: Google utilizes small LLMs to power its search engine. These models understand search queries' semantics and generate relevant results.
2. OpenAI: OpenAI deploys small LLMs to fuel its chatbot, Bard, which holds conversations on diverse topics and creates different creative text formats.
3. Hugging Face: Hugging Face provides tools and resources for developers interested in small LLMs. Its library of pre-trained models can be applied across numerous applications.
As small LLMs continue to evolve, there are potential opportunities for innovation beyond chatbots, educational apps, creative applications, research, and business intelligence:
1. Personalized Medicine: Small LLMs could underpin personalized medicine applications, analyzing patient data and generating customized treatment plans, improving treatment efficacy and minimizing side effects.
2. Virtual Assistants: We could see more engaging, natural virtual assistants developed using small LLMs, assisting users in tasks like appointment scheduling, reminders, and travel arrangements.
3. Social Media: Small LLMs could refine our social media interactions by filtering spam and hate speech, generating engaging content, and aiding users in connecting with like-minded individuals.
4. Security: Small LLMs could enhance computer systems' security by detecting malware, identifying phishing attacks, and preventing data breaches.
5. Transportation: Small LLMs could improve travel experiences by optimizing traffic flow, providing personalized routing suggestions, and delivering real-time travel condition updates.
With the rise of small LLMs comes a need for a serious discussion about AI safety and regulation. The increasing power and capabilities of these models indicate potential use in applications with substantial societal impact. Concerns are growing regarding the possibility of malicious use, such as spreading misinformation or generating harmful content.
While small LLMs are still being developed, potential risks, like biases, inaccuracies, and the generation of offensive content, remain a concern. The widespread accessibility of these models also presents a risk if used by individuals who may not fully understand the associated hazards or lack the expertise for safe usage.
This underscores the need for discussions around ensuring safe and responsible development and usage of small LLMs. Key issues under consideration include:
While there is no one-size-fits-all solution to these challenges, open and transparent discussions can help ensure that small LLMs are utilized beneficially rather than harmfully.
Below are some examples of potential misuse of small LLMs:
While these represent only a few of the many potential misuses of small LLMs, being aware of these risks and implementing mitigating steps is crucial.
The advent of small LLMs marks a significant shift in the AI realm. Being more efficient and accessible than their larger counterparts, small LLMs can pave the way for novel and innovative AI applications.
In the forthcoming years, small LLMs could profoundly influence a variety of industries. Here are some potential impacts:
Besides these specific applications, small LLMs might also generally alter our interactions with computers. They could be harnessed to design more natural and engaging user interfaces, thereby simplifying computer usage and enhancing the overall user experience.
Indeed, the emergence of small LLMs represents a significant development with the potential to revolutionize computer interaction, learning, and decision-making. As these models evolve, we can anticipate even more innovative and exciting applications.
Let's delve into some specific predictions about the future of small LLMs:
However, it's crucial to remember that these are merely predictions, and the future of small LLMs remains uncertain. Yet, it is undeniable that these models hold great potential to significantly influence the AI landscape.
Conclusion and Call to Action
In this article, we explored the impact of AI's democratization on various sectors, the potential it unleashes for individual developers, startups and medium-sized businesses, and how it's reshaping the landscape of AI and technology at large.
For those interested in going deeper into the subject of AI democratization and Large Language Models, here are some key readings that can provide further insights:
If you're an LLM developer or working with AI, we urge you to share your expertise at GIDS. We invite you to propose a talk to the DeepTech track at GIDS 2024: https://form.jotform.com/developersummit/gids-2024-call-for-proposals. Let's shape AI's future together.
Have questions or comments about this article? Reach out to us here.
Banner Image Credits: Inrupt's John Bruce at Great International Developer Summit
“Once again Saltmarch has knocked it out of the park with interesting speakers, engaging content and challenging ideas. No jetlag fog at all, which counts for how interesting the whole thing was."
Cybersecurity Lead, PwC
“Very much looking forward to next year. I will be keeping my eye out for the date so I can make sure I lock it in my calendar."
Software Engineering Specialist, Intuit
“Best conference I have ever been to with lots of insights and information on next generation technologies and those that are the need of the hour."
Software Architect, GroupOn
“Happy to meet everyone who came from near and far. Glad to know you've discovered some great lessons here, and glad you joined us for all the discoveries great and small."
Web Architect & Principal Engineer, Scott Davis
“Wonderful set of conferences, well organized, fantastic speakers, and an amazingly interactive set of audience. Thanks for having me at the events!"
Founder of Agile Developer Inc., Dr. Venkat Subramaniam
“What a buzz! The events have been instrumental in bringing the whole software community together. There has been something for everyone from developers to architects to business to vendors. Thanks everyone!"
Voltaire Yap, Global Events Manager, Oracle Corp.