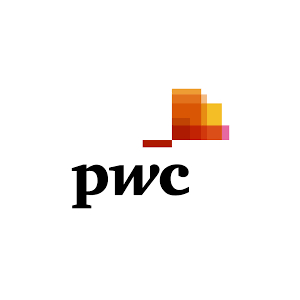
“Once again Saltmarch has knocked it out of the park with interesting speakers, engaging content and challenging ideas. No jetlag fog at all, which counts for how interesting the whole thing was."
Cybersecurity Lead, PwC
Exploring the Data Lakehouse: A comprehensive guide navigating the evolution of data architecture, understanding the benefits and challenges of the data lakehouse, and shedding light on its implementation through real-world examples.
Data architecture is experiencing rapid evolution, catering to organizations' increasing desire to effectively use data for informed decision-making. Traditionally, data warehouses were the go-to storage and analysis solution, yet they faltered when dealing with vast volumes of unstructured data, proved expensive to maintain, and faced challenges with data updates.
As a response, data lakes surfaced, equipped to store all data types in their raw format, offering greater scalability, flexibility, and cost-efficiency. But, they stumbled when it came to querying, analysis, data governance, and security. Enter the data lakehouse - a hybrid data platform marrying the best of data warehouses and lakes.
Major players in the data lakehouse sphere include Amazon Redshift Spectrum, Google Cloud BigQuery, Microsoft Azure Synapse Analytics, Snowflake and Databricks. The growing volume and variety of data, demand for real-time analytics, and need for agile data platforms are propelling data lakehouses to the forefront of data architecture.
Data lakehouses merge the flexibility, cost-effectiveness, and scalability of data lakes with the governance and ACID transactions of data warehouses. They create a single repository for all data types, ensuring accessibility for various tools and applications.
Here's a snapshot of a data lakehouse's structure and functions:
Data lakehouses present a tempting proposition for organizations due to their scalability, flexibility, and cost-efficiency.
Further, lakehouses boost data quality, expedite decision-making, and foster innovation by offering a single truth source for data.
To illustrate, let's consider a few real-world examples:
As the data volume and variety expand, lakehouses will play a pivotal role in enabling data-driven insights and decisions for organizations.
While data lakehouses promise numerous benefits, they pose certain challenges that organizations must consider:
Additional obstacles may include a shortage of skilled professionals, poor data quality, and potential data silos. Despite these challenges, with careful consideration, a lakehouse can prove beneficial.
While data lakehouses offer several advantages over traditional data warehouses and data lakes, they also require more resources for implementation and management. A thorough assessment of an organization's needs will help determine the most suitable solution.
When deciding between a data lakehouse, data warehouse, or data lake, organizations should factor in the following considerations:
Evaluating these aspects based on their specific needs will guide organizations in choosing the most beneficial data architecture for their operations.
The ultimate decision of an organization to adopt a data lakehouse, data warehouse, or a data lake will hinge on their unique needs and circumstances. To make an informed decision, organizations should reflect on various factors:
Here are some additional factors that organizations may want to consider:
By carefully considering these factors, organizations can make informed decisions about whether or not a data lakehouse is the right choice for them.
This case is an enlightening example of how businesses can leverage lakehouses for their data management needs.
Company: Capital One
Problem: Capital One was struggling to keep up with the growing volume and variety of data it was generating. The company's data warehouse was not scalable enough to handle the increasing load, and it was difficult to integrate data from different sources.
Solution: Capital One implemented a data lakehouse using Amazon S3 and Redshift. The data lakehouse allowed the company to store all of its data in a single repository, regardless of its format. This made it easier to integrate data from different sources and to analyze the data more effectively.
Impact: The data lakehouse has helped Capital One to improve its decision-making and to better understand its customers. The company has been able to launch new products and services more quickly, and it has been able to reduce fraud more effectively.e
Here are some specific examples of how the data lakehouse has benefited Capital One:
Capital One is just one example of a company that has successfully implemented a data lakehouse. As the volume and variety of data continues to grow, data lakehouses are becoming increasingly popular as a way to store and analyze data.
Join us at the Great International Developer Summit (GIDS) 2024 and take your data engineering skills to new heights. Explore the power of cutting-edge data platforms, tool and technologies that will revolutionize your projects. Buy Tickets and, if you're a seasoned data engineering or data science expert with experience presenting talks at large conferences, Submit Proposals for Talks to be part of this exciting event. Learn more at the GIDS 2024 Official Website.
Have questions or comments about this article? Reach out to us here.
Banner Image Credits: Attendees at Great International Developer Summit
“Once again Saltmarch has knocked it out of the park with interesting speakers, engaging content and challenging ideas. No jetlag fog at all, which counts for how interesting the whole thing was."
Cybersecurity Lead, PwC
“Very much looking forward to next year. I will be keeping my eye out for the date so I can make sure I lock it in my calendar."
Software Engineering Specialist, Intuit
“Best conference I have ever been to with lots of insights and information on next generation technologies and those that are the need of the hour."
Software Architect, GroupOn
“Happy to meet everyone who came from near and far. Glad to know you've discovered some great lessons here, and glad you joined us for all the discoveries great and small."
Web Architect & Principal Engineer, Scott Davis
“Wonderful set of conferences, well organized, fantastic speakers, and an amazingly interactive set of audience. Thanks for having me at the events!"
Founder of Agile Developer Inc., Dr. Venkat Subramaniam
“What a buzz! The events have been instrumental in bringing the whole software community together. There has been something for everyone from developers to architects to business to vendors. Thanks everyone!"
Voltaire Yap, Global Events Manager, Oracle Corp.